Keynote:
Towards Automated Artificial Intelligence via Automated Algorithm Selection
Heike Trautmann (Paderborn University, Germany)
Robust Automated Algorithm Selection is of crucial importance for constructing high-performing automated AI systems. It has long been observed that for practically any computational problem that has been intensely studied, different problem instances are best solved using different algorithms. This is particularly pronounced for computationally hard problems, where in most cases, no single algorithm defines the state of the art; instead, there is a set of algorithms with complementary strengths. This performance complementarity can be exploited in various ways, one of which is based on the idea of selecting, from a set of given algorithms, for each problem instance to be solved the one expected to perform best. The task of automatically selecting an algorithm from a given set is known as the per-instance algorithm selection problem and has been intensely studied over the past 15 years, leading to major improvements in the state of the art in single-objective continuous black-box optimisation and in solving a growing number of discrete combinatorial optimisation tasks, including traveling salesperson problems.
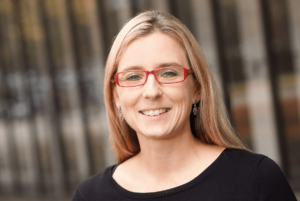
Heike Trautmann is Professor of Machine Learning and Optimization at Paderborn University, Germany. Her research mainly focuses on (Trustworthy) Artificial Intelligence, Machine Learning, Data Science, Automated Algorithm Selection and Configuration, Exploratory Landscape Analysis, (Multiobjective) Evolutionary Optimisation and Data Stream Mining. She is also (Guest) Professor of Data Science in the Data Management and Biometrics group at the University of Twente, Netherlands. Moreover, she serves as key supporter of the Confederation of Laboratories for Artificial Intelligence Research in Europe (CLAIRE), founder and member of the Configuration and Selection of Algorithms (Coseal) Network and Member of the European Research Center for Information Systems (ERCIS) leading the AI and Data Science research cluster.
Keynote:
Recent Advances in Graph Neural Networks:
Concepts, Applications, and Future Directions
Ting Zhong (University of Electronic Science and Technology of China, China)
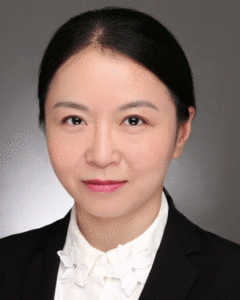
Ting Zhong received the B.S. degree in computer application and the M.S. degree in computer software and theory from Beijing Normal University, Beijing, China, in 1999 and 2002, respectively, and the Ph.D. degree in information and communication engineering from the University of Electronic Science and Technology of China (UESTC), Chengdu, China, in 2009.,She is a Full Professor with UESTC. Her current research interests include deep learning, social networks, and cloud computing.
Keynote:
Empowering Proactive Data Mining Strategies through Generative AI
Teddy Mantoro (Sampoerna University, Indonesia)
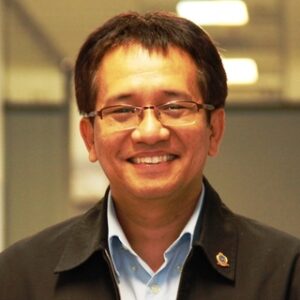